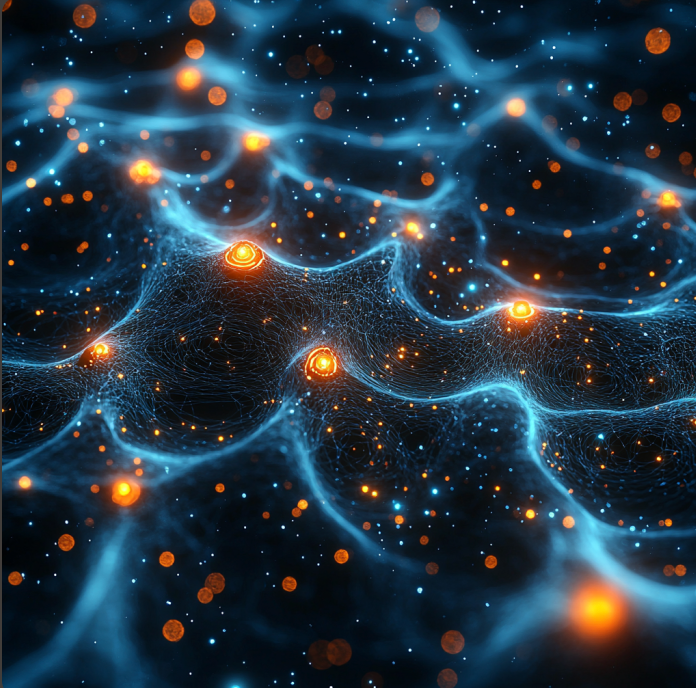
Agentic AI Architecture offers systems the ability to act autonomously and achieve specific goals. This approach is transforming industries by introducing AI systems that are goal-oriented, adaptive, collaborative, and proficient in multi-step problem-solving. Each application of agentic AI architecture is distinct and tailored to a specific niche.
"As agentic AI systems are developed and implemented, we will witness transformative advancements in industry-wide autonomy, customized for specific niches, empowering organizations to scale and optimize their operations with precision," says Priscilla Nuñez, Digital Transformation Executive renowned as The AI Manager, creator of Prisi.la the Multi-Role AI Agent, and Manage the AI •Generative AI Affiliates® GPTs.
How can we fully understand Agentic AI Architecture?
Agentic AI architecture is a framework that enables AI systems to operate independently, making decisions and executing actions without human intervention. These systems are designed to pursue specific objectives, learn from their experiences, and adapt to changing environments.
Key Features of Agentic AI Systems
Goal-Oriented Behavior: Agentic AI systems are programmed to achieve defined objectives, formulating and executing plans to reach desired outcomes.
Adaptability: These systems can adjust their actions based on real-time data and evolving circumstances, ensuring optimal performance in dynamic environments.
Collaborative Functionality: Agentic AI can interact with other agents and tools, facilitating teamwork to accomplish complex tasks.
Proficiency in Multi-Step Problem Solving: They can devise and implement strategies to tackle intricate challenges, breaking them down into manageable steps.
We invite you to contact us for expert guidance in selecting the most suitable strategic framework tailored to your company's needs and to explore how we can assist in enhancing your business management practices.
Implementing agentic AI systems requires a comprehensive approach to ensure their effectiveness and alignment with organizational objectives. The initial step involves defining clear goals and scope for the AI system, ensuring it is designed with a specific purpose in mind. Subsequently, gathering and preprocessing high-quality, relevant data is crucial, as agentic AI thrives on diverse datasets that reflect the environment in which the AI will operate. Selecting appropriate algorithms and designing a modular architecture where components such as perception, reasoning, memory, and action interact seamlessly is essential for building a robust system. Training the agent using supervised, unsupervised, or reinforcement learning methods, incorporating simulations and real-world data, further enhances its capabilities. Implementing feedback loops allows the system to learn from its actions and improve over time. Though most agents are created with autonomy in mind, deploying the agent and continuously monitoring its performance ensures it operates as intended and adapts to any changes in its environment. Adhering to these steps facilitates the development of agentic AI systems that are both effective and aligned with organizational goals.
Agentic AI systems operate through a structured process that enables autonomous problem-solving and decision-making. This process can be delineated into four primary stages:
Perception: In this initial phase, AI agents collect and analyze data from diverse sources, including sensors, databases, and digital interfaces. This involves identifying pertinent features, recognizing objects, and discerning relevant entities within their environment. Such perceptual capabilities are foundational for understanding and interacting with the surrounding world.
Source: NVIDIA Blog
Reasoning: Following data acquisition, the AI employs advanced reasoning mechanisms to comprehend tasks and generate solutions. This involves orchestrating specialized models tailored for functions like content creation, visual processing, or recommendation systems. Techniques such as retrieval-augmented generation (RAG) are utilized to access proprietary data sources, ensuring the delivery of accurate and contextually relevant outputs.
Source: Moveworks: The Copilot for All Employees
Action: Equipped with formulated plans, agentic AI systems integrate with external tools and software through application programming interfaces (APIs) to execute tasks efficiently. Implementing guardrails within these agents ensures tasks are performed correctly and within predefined parameters. For instance, a customer service AI agent might autonomously process claims up to a certain amount, while deferring higher-value claims for human approval.
Source: IBM - United States
Learning: A critical component of agentic AI is its capacity for continuous improvement. Through a feedback loop, often referred to as a "data flywheel," the system assimilates data generated from its interactions. This iterative learning process enhances the models' performance over time, enabling the AI to adapt to new information and evolving circumstances, thereby driving better decision-making and operational efficiency.
Source: Bain & Company
Disclaimer: This article is intended for informational purposes only and should not be considered advice. Portions of the content were generated by AI; however, the author has provided original contributions, including personal perspectives, and has reviewed, edited, and approved all content to ensure its accuracy and coherence. The author reserves the right to update the article and its information at any time.